How Banks Utilize Machine Learning For Fraud Detection

We live in a world where digital transactions are rapidly growing. They have many advantages, but fraudsters are also turning digital, posing serious challenges to financial systems. FTC report says that people lost 10 billion dollars in 2023 alone. This report shows that banks must prioritize protecting customer accounts and economic data. This is possible with the help of machine learning fraud detection in banks.
Machine learning helps banks avoid fraud by identifying unusual patterns in transaction data. This may indicate unauthorized activities and help you mitigate fraud activities in the financial system. Throughout this blog, we dive deep into ML fraud detection in banking, explore types of machine learning models used in fraud prevention, discuss the benefits of adopting this technology, and much more. Let's get started.
What Is Machine Learning?
Machine learning is a branch of AI that helps systems learn from data and recognize patterns of unusual activities. It is necessary to make decisions with minimal human intervention. Machine learning algorithms analyze large data, identify trends, and refine accuracy, unlike humans. This self-improving capability makes ML valuable for tasks that involve large amounts of data and complex patterns.
Machine learning fraud detection is a powerful tool banks can use. A bank may handle millions of transactions daily, and identifying fraud manually is nearly impossible. AI-based and ML technology allows banks to automatically monitor transactions in real-time and flag any unusual patterns that might indicate fraud. It can identify regular customer activity and potential fraud, helping banks respond more swiftly to suspicious transactions.
Traditional Vs. Machine Learning Methods Of Fraud Detection
Fraud detection is crucial to safeguarding customer funds and maintaining trust. Traditionally, banks have used rule-based systems to detect fraudulent activities. As fraudsters' techniques become more sophisticated, your banking system should use technologies like machine learning to make effective solutions.
Traditional Fraud Detection
- Traditional methods use predefined rules to identify suspicious transactions. They use fixed parameters, such as flagging transactions over a certain amount, monitoring activity from unusual locations, or detecting rapid, consecutive transactions.
- This method is easy to implement but has several limitations. Fraudsters are using better technologies, so the traditional method challenges banks in responding to new types of problems without frequently updating the rules.
Machine Learning Fraud Detection
- Many experts consider machine learning a powerful tool for fraud detection as it can analyze large datasets. It can analyze large datasets, recognize patterns, and detect complex fraud behaviors that rule-based systems might miss.
- These algorithms learn from past data and real-time activity, helping you identify unusual activities. For instance, machine learning models can detect unusual activity that deviates from a customer's spending habits and alert banks to potential fraud.
Now that we've covered the basics, let's jump into the exciting part—how different machine-learning techniques can spot sneaky fraudsters!
Types Of Machine Learning Techniques Used In Fraud Detection
Machine learning uses diverse approaches to identify and prevent fraudulent activity. Each technique has unique strengths, and it helps your bank detect fraud in real-time and adapt to emerging threats.
- Supervised Learning: This method is one of the most widely used techniques in fraud detection. Here, the model will get training on labeled data, where each data point is tagged as "fraudulent" or "legitimate." By learning patterns from this labeled dataset, the model differentiates new transactions as likely fraud or normal. Supervised learning is effective for detecting known types of fraud.
- Unsupervised Learning: Unsupervised learning works without labeled data. Instead, it detects anomalies or outliers that deviate from typical transaction patterns and signals the right person with potential fraud. It is valuable for identifying new or unusual types of fraud where there may be little or no labeled data available.
- Semi-Supervised Learning: The semi-supervised method combines supervised and unsupervised learning. This method works well when a small amount of labeled data is available, which is common in fraud detection due to limited verified examples. By mixing the learning method, the model learns from known fraud patterns and explores unknown transaction types. This may improve fraud detection accuracy, especially in scenarios with limited labeled data.
- Reinforcement Learning: In this learning method, the ML uses an adaptive approach, where the model learns through feedback and refines its strategies in the future. In fraud detection, reinforcement learning algorithms receive a reward signal when they correctly identify fraud and a penalty when they miss fraudulent activity or generate false positives. This method continuously improves by learning which patterns or actions lead to successful fraud detection.
Providers like AiPrise integrate these machine-learning techniques to improve your fraud detection systems and improve real-time monitoring capabilities.
Ready to see how you apply machine learning in fraud detection? Let's see that now.
How Can You Apply Machine Learning Fraud Detection In Bank?
Machine learning fraud detection for banks provides advanced tools and techniques that improve security and streamline your banking system. Many companies and financial institutions use this as it gives proactive results. Let's see some application methods now.
- Predictive Analytics For Swift Prevention: This analytics allows your financial institution to identify fraud before it occurs by analyzing historical data and recognizing patterns. By using this model, you can forecast, mitigate the risks, improve security, and reduce financial losses.
- Real-Time Monitoring: Certain real-time monitoring assists banks in assessing transactions when they occur so they can take immediate action on suspicious activities. This quick-response technology assists financial institutions to halt suspicious transactions and protect customer accounts immediately.
- Anomaly Detection In Customer Behavior: Machine learning is a technology that identifies deviations from normal customer behavior, which can signal fraudulent activities. By recognizing patterns, you can detect fraud, respond accordingly, and ensure customers' money is safe.
- Identity Verification By Using Biometrics: Biometric authentication, like facial recognition and fingerprint methods, improves security measures in the identity verification process. When a bank integrates machine learning with biometrics, it allows you to verify identities more accurately.
Want to know more about biometric verification? Read: What Is Biometric Verification?
Would you like to see how this system can help you in the banking system? Let's learn that now.
Benefits Of AI Based Fraud Detection In Banking
The arrival of machine learning has brought a new level of efficiency and effectiveness to fraud detection in banking. Through this AI method, banks can improve security and reduce the burden on traditional systems. Here's how this method helps you in fraud detection:
- Automated Detection: The traditional method requires manual intervention, but this one is completely automatic. Machine learning assesses vast amounts of data in real-time and alerts bank systems instantly. Banks can detect and respond to fraudulent activity better than manual review processes with this method.
Businesses utilizing AiPrise may improve anomaly detection efficiency, potentially reducing the need for human intervention.
- High Accuracy: Machine learning can accurately surpass traditional rule-based systems. After analyzing complex patterns and historical data, these algorithms learn to distinguish between normal and suspicious activities, reduce false positives, and improve detection rates. This means there are fewer chances to flag incorrectly, creating a smoother customer experience.
- Scalability To Handle Large Transaction Volumes: As banks manage millions of transactions daily, machine learning's scalability becomes essential. Machine learning systems can easily handle large transaction volumes, processing and analyzing data without slowing down or compromising accuracy. The scalability of ML ensures that banks can detect fraud effectively as their transaction volume grows.
- Adaptable: The ML models can adapt according to the situation by learning from new data and adjusting their parameters over time. This self-learning ability allows your ML to keep up with emerging threats. With this adaptable method, machine learning helps banks proactively defend against ever-changing fraud tactics.
Benefits At A Glance
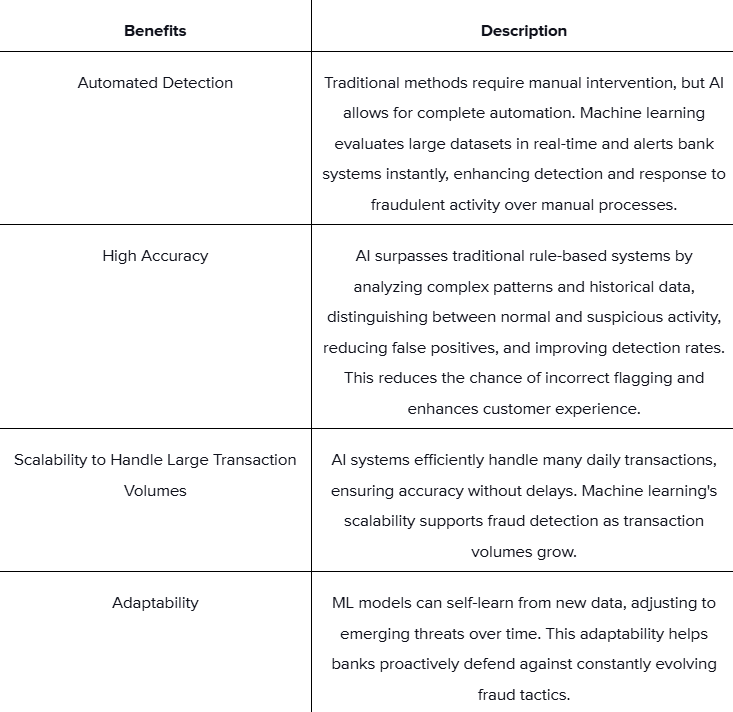
In the following section, let's explore the types of banking fraud tackled by ML.
Types Of Banking Fraud Tackled By Machine Learning
Machine learning addresses various fraud challenges within the financial sector. From detecting unauthorized access to verifying documents, this AI-based technology can address some of the most common and damaging forms of fraud.
1. Prevent And Detect Identity Theft
Identity theft is a common concern for banks because fraudsters may attempt to impersonate customers to access accounts or open new lines of credit. The ML helps you prevent identity theft by analyzing behavioral patterns and comparing them to known profiles.
For instance, Machine learning models enhance the ability to flag suspicious activities by learning complex behavioral patterns that traditional systems may miss. This allows banks to verify users' identities before fraudulent transactions occur.
2. Mitigate Phishing Attacks
Phishing attacks involve tricking individuals into sharing sensitive information. They mostly happen through deceptive emails or messages. Machine learning algorithms analyze communication patterns and detect anomalies in messages that could indicate phishing attempts.
It can scan for suspicious language, links, or attachments and help banks identify and block phishing attempts before they reach customers or internal teams. This early identification is important as it reduces the likelihood of successful attacks.
3. Credit Card Theft Detection
Credit card fraud activities can happen in many ways, such as unauthorized purchases or account takeovers. Machine learning for banks can monitor real-time transactions and detect unusual spending patterns, such as sudden large purchases or transactions from unfamiliar locations.
When models find such things, the banks can quickly identify suspicious credit card activity and take immediate action. You can minimize potential losses by temporarily freezing the card or notifying the customer.
4. Identification Of Document Forgery
There is also a chance that fraudsters alter documents to gain unauthorized access or approval, which is a big challenge for backs. Machine learning fraud detection in banks can analyze documents, such as font consistency, digital signatures, and file metadata, to detect signs of tampering.
The ML scans even minor document inconsistencies and helps you verify document authenticity. As a bank, you can prevent fraudulent activities like this linked to forged paperwork.
We've seen the various types of banking fraud. Now, let's explore the challenges of using machine learning for fraud detection and how we can tackle them!
What Challenges Machine Learning For Fraud Detection Face?
While machine learning offers significant advantages in fraud detection, it also faces several challenges that banks must address during implementation. Understanding these challenges can optimize machine learning models and improve their performance in combating fraud activities.
Handling Inadequate And Unbalanced Datasets
Inadequate and unbalanced datasets can lead machine learning models to produce inaccurate results in detecting fraud.
- The Problem Of Data Scarcity: In many cases, fraud is rarer than legitimate transactions. This results in a dataset not representing the spectrum of activities a model needs to analyze. This imbalance can affect the model's ability to learn effectively, leading to poor detection rates.
- Solutions For Data Imbalance: A bank can use data augmentation or synthetic data generation techniques to create a more balanced dataset. Additionally, ensemble learning methods can improve model performance by combining the strengths of multiple algorithms.
Time-Consuming Training Phases
The training phases for ML can be lengthy and resource-intensive, impacting deployment timelines.
- Lengthy Model Development: Training machine learning fraud detection in a bank can be time-consuming, especially when dealing with large volumes of data. Extensive preprocessing, feature selection, and hyperparameter tuning can prolong the development cycle.
- Efficiency Improvement Strategies: To reduce this challenge, banks can use automated machine learning (AutoML) tools that streamline the training process. With this tool, your business can reduce the time required to train models and bring them to work more efficiently.
Managing False Positives
False positives are a big challenge in maintaining a seamless customer experience and operational efficiency.
- The Impact Of False Positives: False positives happen when legitimate transactions are incorrectly flagged as fraudulent. This may lead to customer dissatisfaction and operational inefficiencies. It is important to manage this as it is critical to maintain trust and ensure a smooth customer experience.
- Solution For False Positives: A company can implement advanced techniques, such as anomaly detection and context-aware systems, to avoid such false positives. By refining the model's decision thresholds and incorporating additional customer context, banks can enhance accuracy and minimize disruptions for genuine transactions.
Adapting To Evolving Fraud Techniques
The dynamic nature of fraud activities requires machine learning models to be agile and adaptable in their detection capabilities.
- The Dynamic Nature of Fraud: Fraud tactics are changing, which presents a challenge for machine learning models. As criminals develop new methods to bypass detection systems, the effectiveness of existing models can diminish over time.
- Continuous Learning Approaches: If your business wants to address this, adopt continuous learning approaches that allow models to update and adapt in real-time. By retraining models with new data, financial institutions can improve security against emerging fraud techniques and maintain effective detection capabilities.
Fortunately, AiPrise offers tailored solutions that address these challenges, continuously updating models to meet emerging threats.
Now that we're clear on the challenges and strategies, let's see what you need to remember while implementing machine learning for fraud detection in your bank!
What You Should Do While Implementing Machine Learning In Fraud Detection?
As a bank, you need to maximize the effectiveness of machine learning in fraud detection to get the best result. These strategies ensure that your machine learning models stay accurate, responsive, and secure in the fight against financial fraud.
- Consolidate Data Beforehand: Consolidate data from multiple sources before implementing this model. Effective fraud detection relies on analyzing various data points, from transaction histories to customer behavior patterns. With these data, your financial institution can provide machine learning models with comprehensive input, which improves the models' accuracy in identifying suspicious activity.
- Auditing And Model Updates: The people behind this fraud will improve their technology to break your security system, so machine learning models must be continuously audited and updated. Regular audits help detect any weaknesses or biases in ML, while updates ensure that the models are prepared for new fraud techniques.
- Create A Comprehensive Fraud Risk Profile: Develop a detailed fraud risk profile for each customer or transaction type to increase the model's ability to detect fraud. Understand the unique risks associated with different transaction types, locations, or customer behaviors and fine-tune the models for higher sensitivity to potential fraud.
Collaborating with experts like AiPrise ensures that your implementation is both effective and future-proof against evolving fraud techniques.
- Educate Users On Fraud Risks And Protection: Machine learning fraud detection bank tools are powerful, but human awareness and vigilance are crucial to preventing fraud. Educate your customers and employees about fraud risks and common red flags. Users can support banks' efforts to secure accounts and transactions When they understand how to recognize suspicious activities and protect sensitive information.
Prevent Banking Fraud With Aiprise Advanced Technology
The rise in fraud activities demands equally advanced solutions, and AiPrise delivers with its cutting-edge technology. By using our AI-powered tools and machine learning, banks can now detect and prevent these illegal activities with accuracy and speed. Our tool analyzes customers' transaction patterns and monitors customer behavior, protecting against various types of fraud, such as identity theft, phishing, and card fraud.
Click here to book a demo with AiPrise experts and discuss how our solution can help you prevent fraud.